Daman Bareiss successfully defended his Ph.D.! Congrats Daman! Daman is now off to work with Omron Adept Technologies, Inc.
Model-Based Collision Avoidance for Dynamic Single- and Multi-Robot Systems: Theory and Application in Ground and Aerial Robots
Committee Members: Dr. Kam K. Leang (Chair), Dr. Jur van den Berg, Dr. Jake Abbott, Dr. David Johnson, Dr. Katia Estabridis
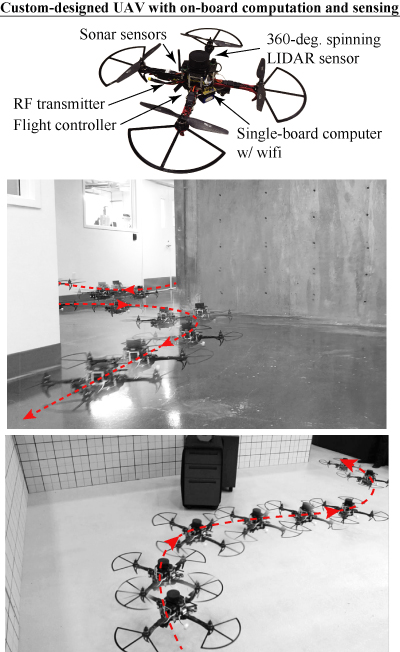
Custom-designed UAV running collision avoidance algorithm and experimental results showing UAV (top) flying through “S”-shaped hallway and (bottom) avoiding obstacles.
Summary: This dissertation solves the collision avoidance problem for single and multi-robot systems where dynamic effects are significant. In many robotic systems (e.g., highly maneuverable and agile unmanned aerial vehicles) the dynamics cannot be ignored and collision avoidance schemes based on kinematic models can result in collisions or provide limited performance, especially at high operating speeds. Herein, real-time, model-based collision avoidance algorithms that explicitly consider the robots’ dynamics and perform real-time input changes to alter the trajectory and steer the robot away from potential collisions are developed, implemented, and verified in simulations and physical experiments. Such algorithms are critical in applications where a high degree of autonomy and performance are needed, for example in robot-assisted first response where aerial and/or mobile ground robots are required to maneuver quickly through cluttered and dangerous environments in search of survivors. Firstly, the research extends reciprocal collision avoidance to robots with dynamics by unifying previous approaches to reciprocal collision avoidance under a single, generalized representation using control obstacles. In fact, it is shown how velocity obstacles, acceleration velocity obstacles, continuous control obstacles, and linear quadratic regulator (LQR)-obstacles are special instances of the generalized framework. Furthermore, an extension of control obstacles to general reciprocal collision avoidance for non-linear, non-homogeneous systems where the robots may have different state spaces and different non-linear equations of motion from one another is described. Both simulations and physical experiments are provided for a combination of differential-drive, differential-drive with a trailer, and car-like robots to demonstrate that the approach is capable of letting a non-homogeneous group of robots with non-linear equations of motion safely avoid collisions at real-time computation rates. Secondly, the research develops a stochastic collision avoidance algorithm for a tele-operated unmanned aerial vehicle (UAV) that considers uncertainty in the robot’s dynamics model and the obstacles’ position as measured from sensors. The model-based automatic collision avoidance algorithm is implemented on a custom-designed quadcopter UAV system with on-board computation and the sensor data is processed using a split-and-merge segmentation algorithm and an approximate Minkowski difference. Flight tests are conducted to validate the algorithm’s capabilities for providing tele-operated collision-free operation. Finally, a set of human subject studies are performed to quantitatively compare the performance between the model-based algorithm, the basic risk field algorithm (a variant on potential field), and full manual control. The results show that the model-based algorithm performs significantly better than manual control in both the number of collisions and the UAV’s average speed, both of which are extremely vital, for example, for UAV-assisted search and rescue applications. Compared to the potential-field based algorithm, the model-based algorithm allowed the pilot to operate the UAV with higher average speeds.